Global spending on artificial intelligence is forecast to reach $52 billion in 2021, achieving a compound annual growth rate of 46.2% from 2016. How can decision-makers and boards make more intelligent investments and decisions when planning their AI strategy?
That’s the topic of a session scheduled during the World Economic Forum’s annual meeting in Davos, January 22–25, which will explore trade-offs, opportunities and risks associated with AI investments.
Concerns regarding racial or gender bias in AI have arisen in areas such as hiring, policing, judicial sentencing and financial services, so at the session, the Forum is planning to introduce a prototype tool kit to help companies avoid such legal and ethical pitfalls.
“Boards are tasked with oversight of all aspects of the work of the company but particularly strategy and risk,” says Kay Firth-Butterfield, head of AI and Machine Learning at the World Economic Forum’s Center For The Fourth Industrial Revolution. “Therefore, when the C-suite proposes use of AI the board must understand how such use will affect the company for good or ill. In doing so they will need to appreciate that AI is a new type of tool which allows a 360 vision of the business, and appraise the strategy accordingly. Additionally, as AI is being used more we are learning more about things which can go wrong with algorithms. In their risk function members of the board must be able to assess brand and social risks of uses of AI. This tool will help them to make the necessary decisions.”
The tool kit will be available later this year through a website which will be organized in modules:
*A brand module will give advice about both how to protect company image in the eye of the public and build brand reputation by using AI to improve society.
*A competition module will look at how companies might use AI to accomplish their mission, covering areas such as impact on strategy, competition and industries, and using AI to disrupt and compete. It will also talk about foreseeing risk.
*A customers module will focus on how to use AI to strengthen customer relationships by building trust and improving service.
*An operating model will advise companies on how to use AI to improve processes and productivity.
*A people and culture module will look at how AI can be used to augment the work force, for employee engagement and for improving diversity.
*Control modules will cover ethics, governance, risk and audits.
The launch of the tool kit comes at a time when companies are increasingly concerned about the risk of their products being used in ways that are unpredictable and unintended. Salesforce made headlines in December when it hired an “ethical and humane use officer.”
In Davos, “we are going to invite people to gets their hands on the tool kit and then pilot it,” says Firth-Butterfield. BBVA, the large Spanish bank, has already agreed to a pilot and the Forum is hoping a half dozen others will as well.
HR’s Use Of AI Is Growing And So Are Concerns
As part of its work on AI and Bias the Forum is planning on releasing a white paper in February that will specifically focus on guidelines for applying AI to human resources (HR).
Some 78% of HR departments expect to see machine learning in at least one HR process within two years, according to a recent survey conducted by Bain & Company, which polled human resources executives and managers at 500 large companies in the U.S., Germany and the United Kingdom, including publicly traded and private companies across a wide-range of industries from manufacturing to retail to healthcare.
Some companies have already run into problems. Amazon, for example, had to scrap a system it was using because it found it was biased against women candidates. Amazon’s computer models were trained to vet applications by observing patterns in resumes submitted to the company over a 10-year period. Most came from men, a reflection of male dominance across the tech industry.
Since machine learning is based on historical data, biases can easily be baked in. Such institutional patterns of disadvantage, stemming from contemporary and historical legacies such as racism, sexism and unequal economic opportunity, continue to deeply affect the workplace and make the creation of fair and transparent algorithms for hiring very difficult, according to the Forum.
The recognition of a candidate’s abilities and achievements is also contingent on the structures of relations and associations that define positions within organizations, so gender stereotypes may lead some managers to overlook the ability of a female candidate for a specific position.
Potential recommendations include encouraging employers and vendors to actively fight against potential bias at every step of the hiring process: from sourcing through screening and interviewing to selection. This could include prompting human resource officers to ask: How is the job description framed? Who can see this job ad? How do we examine resumes? How do we conduct interviews?
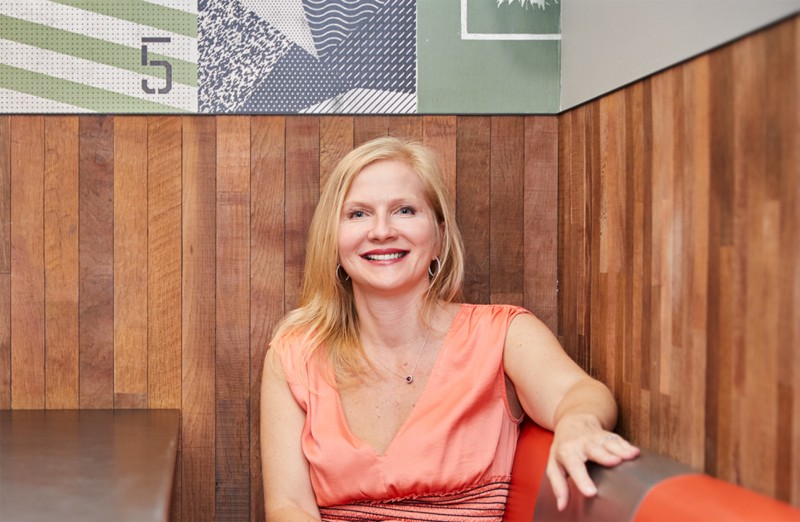
Another issue is that some of the AI systems currently being deployed are opaque, offering little insight into why one candidate is recommended over another. When a company fills a position it should be able to explain the rationale to successful and rejected candidates. “HR professionals should keep in mind that ensuring equal opportunities to all qualified candidates irrespective of their backgrounds is not a classic data science challenge — i.e. finding top talent and good fit by unlocking information contained in data that was previously unusable or inaccessible — but requires questioning of the biased metrics by which algorithms assess ability and potential,” says Firth-Butterfield.
But that doesn’t mean that corporations should shy away from using AI in hiring, says Frida Polli, CEO and co-founder of pymetrics, a 2018 World Economic Forum Technology Pioneer. Technology can be a double-edged sword: the company says that by using neuroscience and artificial intelligence it can predict the right person for the job, while removing bias from the process.
“AI is getting tainted but it is neutral,” says Polli, who has an MBA from Harvard Business School and a PhD in neuropsychology. “It is not good or bad.”
Polli says her company’s technology can pinpoint 80 cognitive and emotional features collected from people in aggregate and use them as predictors of success in different roles.
It works like this: Objective behavioral data is collected using neuroscience exercises in the form of games. Customized, automated machine learning is used to maximize prediction. Pymetrics’ technology methodologically removes bias from algorithms via an algorithm auditing process, which it says ensures a lack of bias. And rejected candidates can automatically match to other opportunities at other companies using pymetrics.
The company, which launched in 2016, has 100 corporate clients, including Unilever, Accenture and Microsoft.
Unilever decided to do all of its hiring through pymetrics and is happy with the results, says Polli. “What they found was that they increased their ethnic representation and also hired from a much more diverse socio-economic group. We know these people would not have been hired otherwise and they have been successful.”
Polli argues that it is easier to audit algorithms than humans, many of whom are hard-wired with biases of their own. “If you are not using a technology there is a much greater risk that your hiring practices are on legal shaky ground,” she says.